大家好,欢迎来到IT知识分享网。
推荐阅读:
- 面试BAT 却被小小字符串秒杀?这13道题帮你一举击败字符串算法题
- 字节跳动秋招面经:后端开发工程师,已拿意向书
前言
平时的编码中,我们经常需要判断两个文本的相似性,不管是用来做文本纠错或者去重等等,那么我们应该以什么维度来判断相似性呢?这些算法又怎么实现呢?这篇文章对常见的计算方式做一个记录。
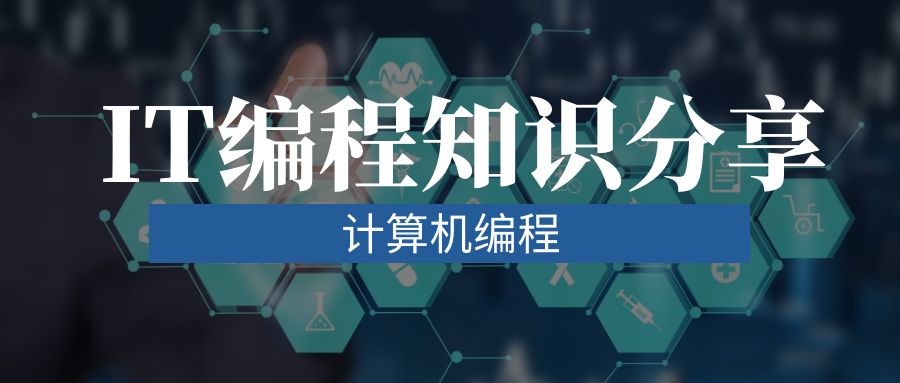
Jaccard 相似度
首先是 Jaccard 相似度系数,下面是它在维基百科上的一个定义及计算公式。
The Jaccard index, also known as Intersection over Union and the Jaccard similarity coefficient (originally given the French name coefficient de communauté by Paul Jaccard), is a statistic used for gauging the similarity and diversity of sample sets. The Jaccard coefficient measures similarity between finite sample sets, and is defined as the size of the intersection divided by the size of the union of the sample sets:
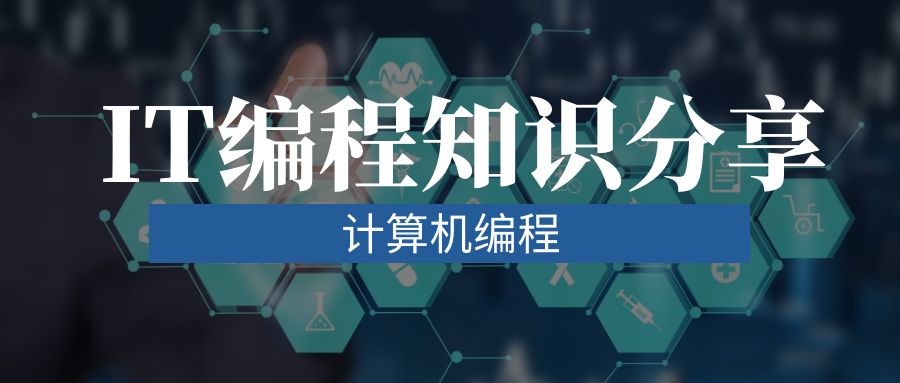
其实总结就是一句话:集合的交集与集合的并集的比例.
java 代码实现如下:
public static float jaccard(String a, String b) { if (a == null && b == null) { return 1f; } // 都为空相似度为 1 if (a == null || b == null) { return 0f; } SetaChar = a.chars().boxed().collect(Collectors.toSet()); Set bChar = b.chars().boxed().collect(Collectors.toSet()); // 交集数量 int intersection = SetUtils.intersection(aChar, bChar).size(); if (intersection == 0) return 0; // 并集数量 int union = SetUtils.union(aChar, bChar).size(); return ((float) intersection) / (float)union; }
Sorensen Dice 相似度系数
与 Jaccard 类似,Dice 系数也是一种计算简单集合之间相似度的一种计算方式。与 Jaccard 不同的是,计算方式略有不同。下面是它的定义。
The Sørensen–Dice coefficient (see below for other names) is a statistic used to gauge the similarity of two samples. It was independently developed by the botanists Thorvald Sørensen[1] and Lee Raymond Dice,[2] who published in 1948 and 1945 respectively.
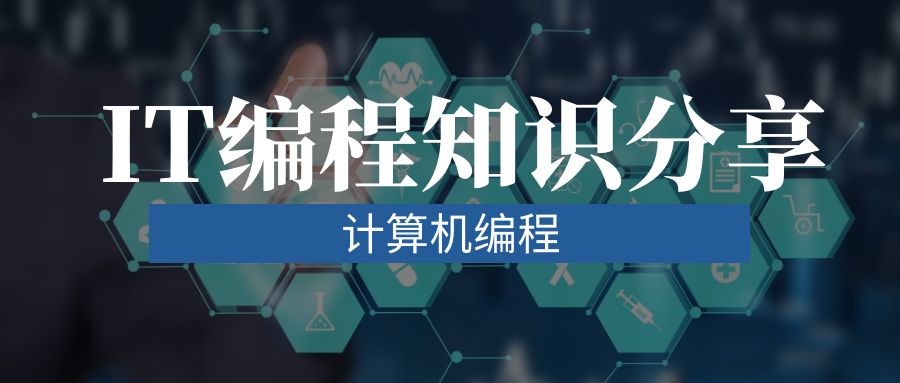
需要注意的是,他是:集合交集的 2 倍除以两个集合相加。并不是并集.
java 代码实现如下:
public static float SorensenDice(String a, String b) { if (a == null && b == null) { return 1f; } if (a == null || b == null) { return 0F; } SetaChars = a.chars().boxed().collect(Collectors.toSet()); Set bChars = b.chars().boxed().collect(Collectors.toSet()); // 求交集数量 int intersect = SetUtils.intersection(aChars, bChars).size(); if (intersect == 0) { return 0F; } // 全集,两个集合直接加起来 int aSize = aChars.size(); int bSize = bChars.size(); return (2 * (float) intersect) / ((float) (aSize + bSize)); }
Levenshtein
莱文斯坦距离,又称 Levenshtein 距离,是编辑距离的一种。指两个字串之间,由一个转成另一个所需的最少编辑操作次数。
简单的说,就是用编辑距离表示字符串相似度, 编辑距离越小,字符串越相似。
java 实现代码如下:
public static float Levenshtein(String a, String b) { if (a == null && b == null) { return 1f; } if (a == null || b == null) { return 0F; } int editDistance = editDis(a, b); return 1 - ((float) editDistance / Math.max(a.length(), b.length())); } private static int editDis(String a, String b) { int aLen = a.length(); int bLen = b.length(); if (aLen == 0) return aLen; if (bLen == 0) return bLen; int[][] v = new int[aLen + 1][bLen + 1]; for (int i = 0; i <= aLen; ++i) { for (int j = 0; j <= bLen; ++j) { if (i == 0) { v[i][j] = j; } else if (j == 0) { v[i][j] = i; } else if (a.charAt(i - 1) == b.charAt(j - 1)) { v[i][j] = v[i - 1][j - 1]; } else { v[i][j] = 1 + Math.min(v[i - 1][j - 1], Math.min(v[i][j - 1], v[i - 1][j])); } } } return v[aLen][bLen]; }
代码中的编辑距离求解使用了经典的动态规划求解法。
我们使用了** 1 – ( 编辑距离 / 两个字符串的最大长度) ** 来表示相似度,这样可以得到符合我们语义的相似度。
汉明距离
汉明距离是编辑距离中的一个特殊情况,仅用来计算两个等长字符串中不一致的字符个数。
因此汉明距离不用考虑添加及删除,只需要对比不同即可,所以实现比较简单。
我们可以用similarity=汉明距离/长度来表示两个字符串的相似度。
java 代码如下:
public static float hamming(String a, String b) { if (a == null || b == null) { return 0f; } if (a.length() != b.length()) { return 0f; } int disCount = 0; for (int i = 0; i < a.length(); i++) { if (a.charAt(i) != b.charAt(i)) { disCount++; } } return (float) disCount / (float) a.length(); }
下面是测试用例:
Assert.assertEquals(0.0f, StringSimilarity.hamming("java 开发", "大过年的干啥"), 0f); Assert.assertEquals(0.f, StringSimilarity.hamming("大过年的吃肉", "大过年的干啥"), 0f);
余弦相似性
首先是余弦相似性的定义:
余弦相似性通过测量两个向量的夹角的余弦值来度量它们之间的相似性。0 度角的余弦值是 1,而其他任何角度的余弦值都不大于 1;并且其最小值是-1。从而两个向量之间的角度的余弦值确定两个向量是否大致指向相同的方向。两个向量有相同的指向时,余弦相似度的值为 1;两个向量夹角为 90°时,余弦相似度的值为 0;两个向量指向完全相反的方向时,余弦相似度的值为-1。这结果是与向量的长度无关的,仅仅与向量的指向方向相关。余弦相似度通常用于正空间,因此给出的值为 0 到 1 之间。
计算公式如下:
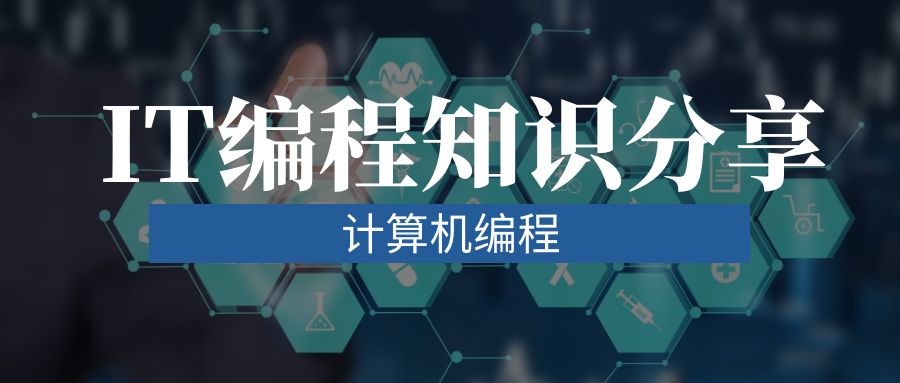
余弦我们都比较熟悉,那么是怎么用它来计算两个字符串之间的相似度呢?
首先我们将字符串向量化,之后就可以在一个平面空间中,求出他们向量之间夹角的余弦值即可。
字符串向量化怎么做呢?我举一个简单的例子:
A: 呼延十二 B: 呼延二十三 他们的并集 [呼,延,二,十,三] 向量就是并集中的每个字符在各自中出现的频率。 A 的向量:[1,1,1,1,0] B 的向量:[1,1,1,1,1] 然后调用上面的公式计算即可。
java 代码实现如下:
public static float cos(String a, String b) { if (a == null || b == null) { return 0F; } SetaChar = a.chars().boxed().collect(Collectors.toSet()); Set bChar = b.chars().boxed().collect(Collectors.toSet()); // 统计字频 Map aMap = new HashMap<>(); Map bMap = new HashMap<>(); for (Integer a1 : aChar) { aMap.put(a1, aMap.getOrDefault(a1, 0) + 1); } for (Integer b1 : bChar) { bMap.put(b1, bMap.getOrDefault(b1, 0) + 1); } // 向量化 Set union = SetUtils.union(aChar, bChar); int[] aVec = new int[union.size()]; int[] bVec = new int[union.size()]; List collect = new ArrayList<>(union); for (int i = 0; i < collect.size(); i++) { aVec[i] = aMap.getOrDefault(collect.get(i), 0); bVec[i] = bMap.getOrDefault(collect.get(i), 0); } // 分别计算三个参数 int p1 = 0; for (int i = 0; i < aVec.length; i++) { p1 += (aVec[i] * bVec[i]); } float p2 = 0f; for (int i : aVec) { p2 += (i * i); } p2 = (float) Math.sqrt(p2); float p3 = 0f; for (int i : bVec) { p3 += (i * i); } p3 = (float) Math.sqrt(p3); return ((float) p1) / (p2 * p3); }
对上面的代码运行了测试用例,可以看到基本符合我们的期望。
Assert.assertEquals(0.f, StringSimilarity.cos("apple", "app"), 0f); Assert.assertEquals(0.f, StringSimilarity.cos("呼延十二", "呼延二十三"), 0f); Assert.assertEquals(0.0f, StringSimilarity.cos("数据工程", "日本旅游"), 0f);
总结
本文简单的介绍了几种不同的计算纯文本之间相似度的方式,他们在一定程度上都是奏效的,但是,各自也有各自的一些含义在里面,比如有的使用编辑距离来描述,有的用向量夹角来描述。所以在使用到本文中的方式时,还是要多多了解他的原理,结合自己的业务实际,选择其中的一种或者几种进行使用。
作者:呼延十
原文链接:https://juejin.im/post/5dca66e0e51d456a2e6556f8
免责声明:本站所有文章内容,图片,视频等均是来源于用户投稿和互联网及文摘转载整编而成,不代表本站观点,不承担相关法律责任。其著作权各归其原作者或其出版社所有。如发现本站有涉嫌抄袭侵权/违法违规的内容,侵犯到您的权益,请在线联系站长,一经查实,本站将立刻删除。 本文来自网络,若有侵权,请联系删除,如若转载,请注明出处:https://yundeesoft.com/52301.html